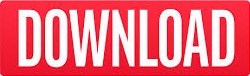
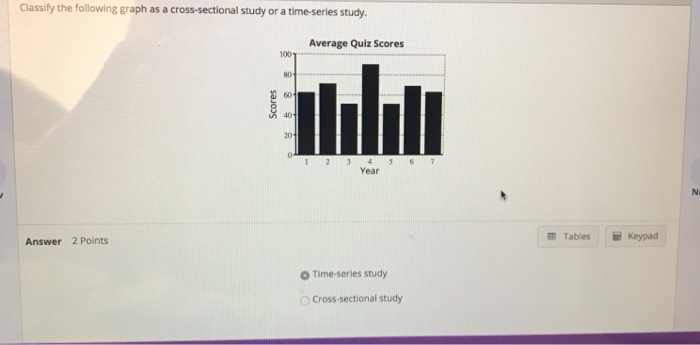
It was time to address the elephant in the room. In total, I wanted to extract data from 31 cities, so multiplying 4 hours by 31 cities equals roughly 5 days of manual downloading. It would take me a total of 253 minutes or over 4 hours of continuous clicking to download all data sets for a single city. Among the available cities, the most amount of days of data is of 3.25 years (January 2016 to March 2020) or 39 months. It took me around 6 minutes and 30 seconds to download data for a single month. I knew automating this process would be much easier, but I didn’t have the coding skills, nor did I know what packages I needed to use for this task. So I thought: “why not click on each day and download its respective data set?”. Uber Movement calendar where you can choose the desired date range. Rather, it spits out a csv file with one single value for the three-month average travel time for each pair of zones. That is, if you select to download values from January 2020 to March 2020, you won’t receive 90 values, which is roughly the amount of days in that range. Whatever date range you are interested in getting travel times for does not consist of daily data. The Uber Movement website allows you to download data from any zone to every other zone in the city. Therefore, I needed to get my hands on daily travel times. The smaller the time increment, the better. The more rows of data I had, the greater the predictive power of my models (potentially). In order to have a more statistically precise outcome, I needed all the data I could get. “Let me just download the travel times and plot some numbers so I can move on to exploratory data analysis (EDA).”Īs it turns out, the data I wanted was not so easy to extract. Sample travel times for my home town, São Paulo.
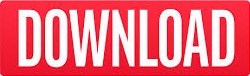